Why Should Legal And Policy Professionals Learn The Data Language?
Archana Sivasubramanian
20 May 2020 7:15 AM
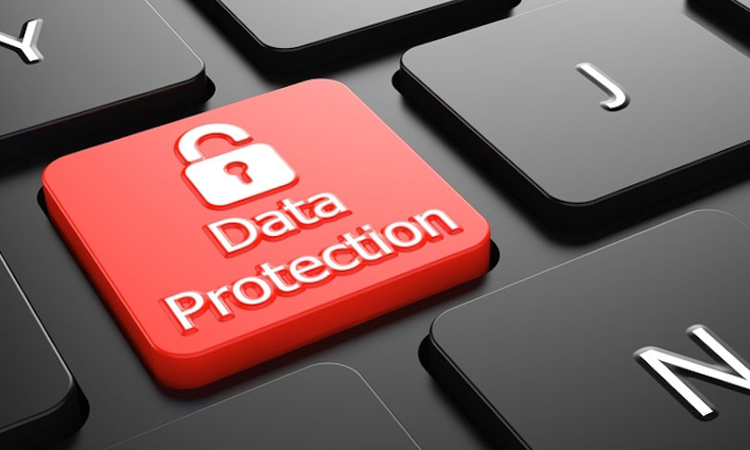
Data has always informed and influenced governments and businesses in making decisions, but this is not a modern trend. This type of decision-making can be traced back to 6CE, where the Herodus Census (Quirinius) was used as a narrative means to establish the birth of Jesus in Bethlehem. Since then, tables of data have been used to make economic and military decisions. Daniel Bernoulli developed the first mathematical model—which he described as a 'new province into the body of mathematics'—and which was used for epidemiology, particularly to defend the practice of inoculation against small pox. His model was heavily criticised, but it also brought to the fore some very interesting debates on the relationship between statistics and causality. The philosopher Voltaire joined this debate in 1772 and wrote a book on probability and decision-making in the legal context, eventually leading to a reform of the French Legal System. And, in the course of the last two centuries since, we have seen more sophisticated data modelling techniques adopted in the decision-making process.
Today, computer intelligence and digitisation have significantly altered the nature and scope of data. Several buzzwords such as 'big data' and 'data-driven models' heavily influence policy decisions, but these terms also remain poorly understood. The data discourse, in fact, hasn't been more relevant in human history. In this unprecedented moment of pandemic, data is being used to mine information, draw up models to predict the disease spread and direct capacity-building in healthcare systems. Be it easing the burden of lockdown slowly or developing tools to soften the economic impact, epidemiological modelling also largely informs our decision-making process to develop policy interventions for COVID-19. Data's omnipresence is not a cognitive bias.
Knowledge of data, evidently so, is crucial to build crisis responses, but are data skills only meant for data scientists? Data-driven decision-making is not a siloed process. It is a thoughtful exercise that enables a collaborative decision-making culture by helping stakeholders see the other side of the fence, a new perspective to understand any problem.
Policy-making is all about making sense of 'what works' but excessive reliance on data also has its own pitfalls. Learning the language of data analysis is crucial to understanding the shifts in the nature of both human and machine decision making, and recognizing the consequences of such data-driven decisions. For this reason alone, looking at data should be first in the order of processes that a lawyer or a bureaucrat or a policy-maker must employ to guide public policy, combat misinformation and handle legal complexities emerging from intricate situations.
There are several benefits to this method of decision-making:
First, a knowledge of data can help deal with uncertainty.
A foundation in statistics leads to two outcomes. It helps a) arrive at some hypotheses about a data-set b) infer the accuracy of the very estimates that have been made. A quantitative data summary may not contain all the information that is required to deal with a situation, but it helps navigate uncertainty to a good measure. Computer models often are very complex, and dynamic but they offer at least one vision of a possible future. Rather than getting stuck in the pervasive psychological effects of uncertainty, through statistics, we can transfer some of our anxieties into actionable knowledge and beautiful data-models. At the very least, there is an illusionary sense of direction where none existed before.
Second, understanding data can help recognise that data models aren't fool-proof.
While data modelling can help us remain less anxious, they also do not have all the answers. They are not the purveyors of truth. Predictive models are bound to produce a wide range of outcomes. These outcomes are not only determined by the quality and quantity of data that has been gathered but also by the number of unknowns, like in the case of COVID-19. Not every data model can be relied upon to be the mainstay of a policy decision. As more evidence comes to light, truth shifts and takes a new silhouette, one data model at a time. And with it, policy response also changes.
The United Kingdom's COVID-19 policy is a case in point. In early March this year, Johnson's government stood alone in pursuing a herd immunity policy drawing on past epidemic models and scientific advice. But subsequent analysis from a team modelling the spread and impact of COVID-19 forced the government to abandon this route and enforce lockdown at the end of the same month. A done-to-death quote by statistician George Box remains a useful rule of thumb to remember during such occasions: 'all models are wrong, but some are useful'.
Third, data knowledge is crucial to evaluate the impact of interventions.
While policy recommendations for COVID-19 are aimed towards 'flattening the curve', there is also a torrent of ambiguity in the decision-making process. This is because data-sets are imperfect and do not help make perfect decisions; but an informed view of data can effect in the quality of interventions and performance negotiations. Of course, experimentation is harder in practice for policy-makers and governments, but our confidence in these models comes from the fact that we are able to measure the different types, intensities and durations of interventions to be implemented, and how these impact the spread of disease over time.
This knowledge will help decision-makers determine the future course of action, and also grasp some underlying principles to guide what sometimes can be a truly wicked problem.
Fourth, working with data necessarily involves accounting for biases.
Biases are way too many. Comforting lies and unpleasant truths can colour one's interpretation of data. Good decision-making with data, hence, involves looking for patterns in data instead of looking for evidence to confirm one's biases. Good decision-making with data also involves recognising the fact that algorithms cannot be exempted from bias. Machine learning models arrive at decisions based on the data they process. The model is only as good as the data that is fed into it and relying only on them to arrive at decisions is dangerous for social policy-making. Policy-makers must question the veracity of data, work with data scientists to arrive at strategies to build an effective data model, and troubleshoot ideas to ensure that a robust ethical infrastructure aids the process of decision making.
With all this in mind, there clearly is a need for legal and policy professionals to move away from their siloed disciplines and embrace interdisciplinary thinking. They need a battery of skillsets to make sense of these situations and an understanding of data stands paramount to help decipher such crises. We also live at a time that calls for serious reflection on the preparedness of our decision-makers to deal with complex situations. The legal and policy education in India follows a conventional curriculum that removes student learning outcomes away from ground reality. Tough times call for tough measures and this pandemic is a reminder that we need to arm our decision-makers with data skillsets for them to effectively function as a public agent or a business leader.
With Fellowships like Daksha foraying into the education landscape with their innovative pedagogy and curriculum design, future lawyers and policy professionals can not only speak the law and write policy recommendations but also supplement their decisions with a memo on data effectiveness.
Archana Sivasubramanian has a background in public policy and works at the Daksha Fellowship, Madras. Her research interests include state capacity; regulation and data governance; public administration reforms. Author's views are personal.